More than ever, AI’s latest models blur the lines between human-made and AI-generated content
A growing range of AI detectors claims to distinguish this fine line between AI and writers.
Is this claim true? How does AI detection work from the inside?
Whether you’re safeguarding your brand’s voice or ensuring academic rigor, here’s a detailed guide about AI detectors’ real accuracy and reliability.
How Do AI Detectors Detect AI Content?
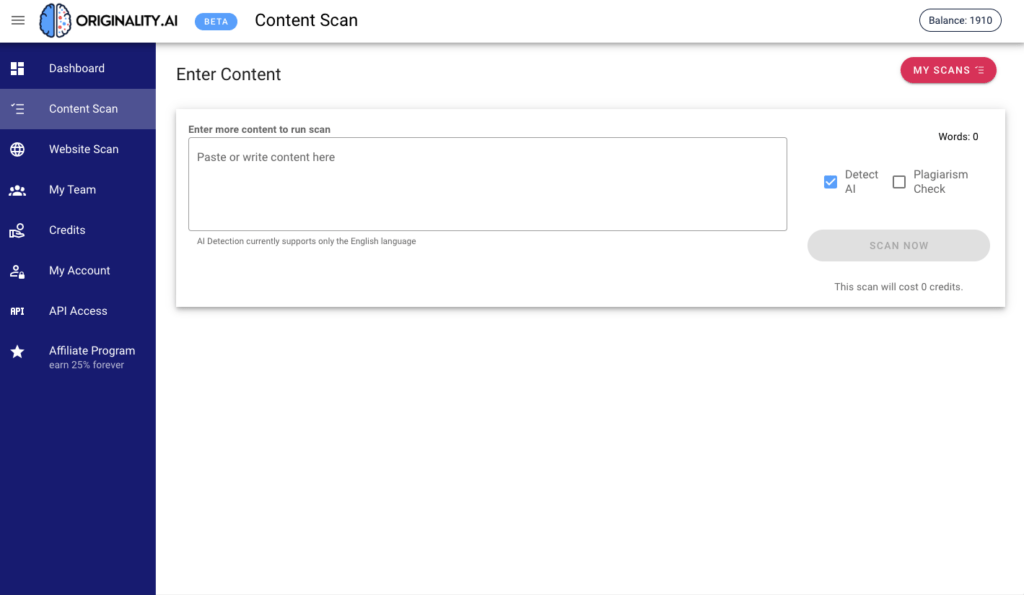
When you use AI detectors, you’re leveraging tools that analyze text via advanced ML and algorithmic techniques.
Here are the characteristics they are looking at :
- Perplexity: This metric gauges how predictable the text is. AI-generated content often scores low on perplexity because it tends to follow predictable patterns. This can make the text sound smooth but sometimes too uniform or bland
- Burstiness: This looks at the variation in sentence length and structure. Your writing might mix short, sharp sentences with longer, more complex ones, showing a high degree of burstiness. AI-generated texts don’t usually have this variety; they stick to a more consistent pattern, which AI detectors pick up as a sign of non-human authorship.
- Statistical Analysis: Many AI detectors compare your text against vast databases of known AI-generated and human-written texts. They look for patterns that are commonly found in AI text, like certain phrase repetitions or simplified syntax.
- Additional Techniques: Some of the most sophisticated detectors analyze grammatical nuances, the coherence of ideas, and even stylistic elements. They assess whether the text flows like something a human would write or if it feels like it was stitched together by a computer.
Do AI Detectors Work?
When you’re relying on AI detectors to check the authenticity of content, you obviously want to be sure about their capabilities and limitations.
Here’s what current research says about their effectiveness:
- Accuracy challenges to edited AI Content: Studies have shown that AI detectors often struggle to maintain high accuracy, particularly when they encounter AI-generated content that has been deliberately modified to avoid detection. In some scenarios, accuracy rates have plummeted to as low as 17.4%.
- Non-english speaker bias: AI detectors can display bias, particularly against content produced by non-native English speakers, often misclassifying it as AI-generated. This can lead to situations where your genuine content is flagged incorrectly.
- False positives and false negatives: AI detector judgment is very inconsistent, sometimes withdrawing altogether from a final judgement by giving an “uncertain” response. They can flag some human-made content as AI and AI-generated content as human, what we also call false positives and false negatives. A false positive, where human-created content is wrongly tagged as AI-generated, can damage reputations and trust, especially in academic settings.
- Impact of model evolutions: the continuous advancement of LLM models makes AI detectors lose accuracy and reliability over time. For example, there’s still a huge gap of accuracy considering the detection of GPT-3,5 compared to GPT-4 and Claude 3. AI detectors need to constantly adapt to stay relevant.
- Formal writing: more formal and structured writing might be more likely to be flagged as AI content by AI detectors. That’s why some tools like Originality.AI warn users to only review more informal writing.
Should You Use AI Detectors for Your Use Case?
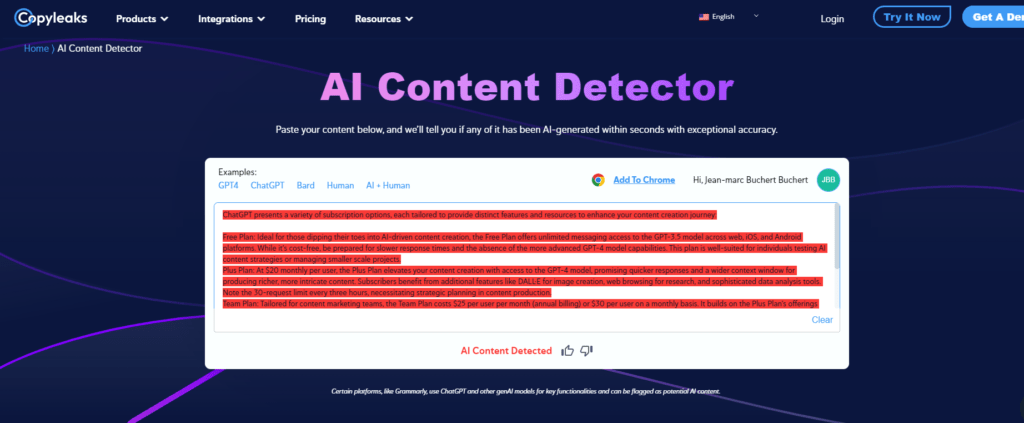
Based on the conclusions drawn from these recent studies, AI detectors come with caveats that demand your attention.
You should first recognize that AI detectors are not infallible. Their effectiveness varies significantly, and some may struggle with accuracy rates when faced with manipulated content designed to evade detection. So they shouldn’t be the sole arbiters in high-stakes environments such as academic settings or professional publishing.
Additionally, the potential for bias in these tools cannot be overlooked. AI detectors have been shown to misclassify content produced by non-native English speakers as AI-generated. This bias complicates matters further when the stakes involve credibility and ethical content management.
Given these limitations, the most effective approach is to use AI detectors as just one part of your broader strategy for verifying content authenticity. These tools should complement, not replace, the nuanced judgments of human reviewers who understand the intricacies of language and context that AI may not fully capture.
If you decide to implement AI detectors, staying updated on the latest developments in AI detection technology is crucial. Regular updates and continuous monitoring of their performance are essential to ensure that the AI detectors remain a useful part of your toolkit.
You also be transparent about how which tool you use and their limitations when making a high-stake judgment.
The Best AI Detectors: A Comparison
When it’s time to choose an AI detection model, you’ll want one that stands out not just in accuracy, but also in not mistaking human writing for AI-generated content.
Latest public and private studies make it possible to have a comparisons of each tool on the market :
Academic studies
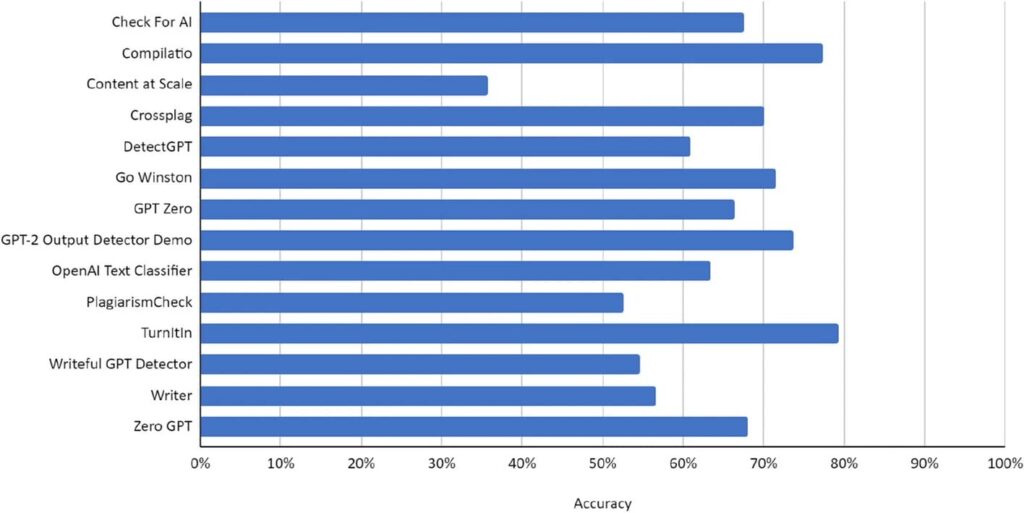
December 2023 study by the International Journal for Educational Integrity collected data from common AI detectors on the market. Here’s how these models rack up
- TurnItIn and OpenAI Text Classifier stand out with the highest accuracy rates, which indicates that they are most likely to correctly identify both AI-generated and human-generated content.
- Crossplag and Compilatio follow closely, suggesting they are reliable options, albeit with a slightly lower accuracy rate than the leaders.
- GPT Zero, Writer, and Go Winston present moderate accuracy levels. This suggests that while they are generally reliable, there may be occasional lapses in correctly identifying content types.
- Content at Scale and Check For AI sit at the lower end of the accuracy spectrum among the tools displayed. They may offer basic detection capabilities, but could potentially benefit from additional verification through other means.
For your strategy, consider the following:
- High-Stakes Content: If your content strategy involves high-stakes information where accuracy is non-negotiable, such as academic writing or technical reports, leaning towards tools like TurnItIn or OpenAI Text Classifier could be beneficial.
- General Content: For more general content, where occasional inaccuracies are less critical, a tool like GPT Zero or Writer might offer a good balance between performance and cost.
- Budget Constraints: If you are working within a tight budget and need a more cost-effective solution, you may consider Content at Scale or Check For AI, keeping in mind that they may require more manual oversight.
Private study
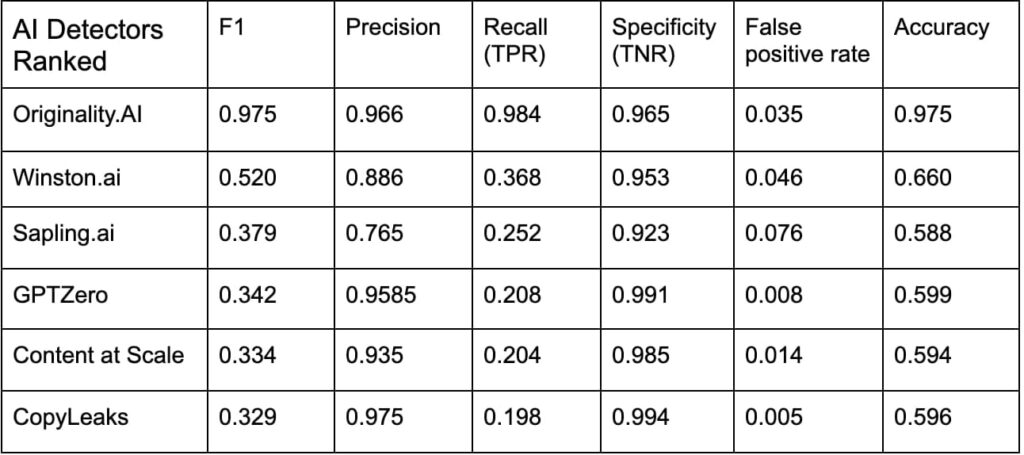
Originally.AI also made its own open-sourced benchmark to compare current AI detection models. While considering the company’s obvious bias, here’s what the mapping is saying :
Originality.AI clearly leads the pack with an F1 score of 0.975. This metric, combining precision and recall, suggests it balances well between identifying AI-generated content and not falsely flagging human-written work. Its precision is stellar at 0.966, meaning when it tags content as AI-generated, it’s right on the mark almost all the time. Plus, with a specificity of 0.965 and an outstanding accuracy of 0.975, you can trust this tool to minimize false positives—only a 0.035 rate—keeping the false alarms to a whisper.
Moving down the line, Winston.ai might give you pause. Despite a decent precision of 0.886, its recall and accuracy tell a different story at 0.368 and 0.660, respectively. It’s more likely to let some AI content slip by, and with a false positive rate of 0.046, it’s not the most reliable for differentiating human text.
Sapling.ai may leave you wanting more with an F1 score of 0.379. Precision isn’t too shabby at 0.765, but a recall of just 0.252 combined with a lower accuracy of 0.588 suggests it might not catch all AI content, and a higher false positive rate of 0.076 means it could ring a few unnecessary alarms.
Now, take a look at GPTZero. It boasts an impressive precision of 0.9585, suggesting it’s trustworthy when it identifies content as AI-generated. However, the low recall of 0.208, paired with an accuracy of just 0.599, implies it might miss a lot of AI-generated content. Its specificity is through the roof at 0.991, so false positives are rare, but is it catching enough?
For Content at Scale, the numbers show a balanced specificity of 0.985 and precision of 0.935. Yet, a recall of 0.204 and accuracy of 0.594 indicate it might not be the best at identifying AI content, even if it rarely mislabels human work.
Lastly, Copyleaks shows promise with a precision of 0.975—when it detects AI content, you can almost bank on it. With the lowest false positive rate at 0.005, it rarely makes a mistake on human content. However, a recall of just 0.198 and accuracy of 0.596 mean it’s not the most effective at catching all AI-written text.
Our conclusion ? Originality.AI seems to shine as the top contender for both accuracy and reliability, while others like GPTZero and Copyleaks excel in precision but may require additional verification due to lower recall.
Time to try all these AI detection tools by yourself, minding their limitations !